GPU621/Apache Spark
Group Members
- Akhil Balachandran
- Daniel Park
vs 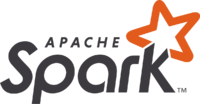
MapReduce was famously used by Google to process massive data sets in parallel on a distributed cluster in order to index the web for accurate and efficient search results. Apache Hadoop, the open-source platform inspired by Google’s early proprietary technology has been one of the most popular big data processing frameworks. However, in recent years its usage has been declining in favor of other increasingly popular technologies, namely Apache Spark.
This project will focus on demonstrating how a particular use case performs in Apache Hadoop versus Apache spark, and how this relates to the rising and waning adoption of Spark and Hadoop respectively. It will compare the advantages of Apache Hadoop versus Apache Spark for certain big data applications.
Introduction
Apache Hadoop
What is Apache Hadoop?
Applications
Apache Spark
Apache Spark is a unified analytics engine for large-scale data processing. It is an open-source, general-purpose cluster-computing framework that provides an interface for programming entire clusters with implicit data parallelism and fault tolerance. Since its inception, Spark has become one of the biggest big data distributed processing frameworks in the world. It can be deployed in a variety of ways, provides high-level APIs in Java, Scala, Python, and R programming languages, and supports SQL, streaming data, machine learning, and graph processing.
Architecture
One of the distinguishing features of Spark is that it processes data in RAM using a concept known as Resilient Distributed Datasets (RDDs) - an immutable distributed collection of objects which can contain any type of Python, Java, or Scala objects, including user-defined classes. Each dataset is divided into logical partitions which may be computed on different nodes of the cluster. Spark's RDDs function as a working set for distributed programs that offer a restricted form of distributed shared memory.
At a fundamental level, an Apache Spark application consists of two main components: a driver, which converts the user's code into multiple tasks that can be distributed across worker nodes, and executors, which run on those nodes and execute the tasks assigned to them.
Components
Spark Core
Spark Core is the basic building block of Spark, which includes all components for job scheduling, performing various memory operations, fault tolerance, task dispatching, basic input/output functionalities, etc.
Spark Streaming
Spart Streaming processes live streams of data. Data generated by various sources is processed at the very instant by Spark Streaming. Data can originate from different sources including Kafka, Kinesis, Flume, Twitter, ZeroMQ, TCP/IP sockets, etc.
Spark SQL
Spark SQL is a component on top of Spark Core that introduced a data abstraction called DataFrames, which provides support for structured and semi-structured data. Spark SQL allows querying data via SQL, as well as via Apache Hive's form of SQL called Hive Query Language (HQL). It also supports data from various sources like parse tables, log files, JSON, etc. Spark SQL allows programmers to combine SQL queries with programmable changes or manipulations supported by RDD in Python, Java, Scala, and R.
GraphX
GraphX is Spark's library for enhancing graphs and enabling graph-parallel computation. It is a distributed graph-processing framework built on top of Spark. Apache Spark includes a number of graph algorithms that help users in simplifying graph analytics.
MLlib (Machine Learning Library)
Spark MLlib is a distributed machine-learning framework on top of Spark Core. It provides various types of ML algorithms including regression, clustering, and classification, which can perform various operations on data to get meaningful insights out of it.
Overview: Spark vs Hadoop
Advantage and Disadvantages
Parallelism
Performance
Analysis: Spark vs Hadoop
Methodology
Setup
Results
Conclusion
Progress
- Nov 9, 2020 - Added project description
- Nov 20, 2020 - Added outline and subsections
- Nov 21, 2020 - Added content about Apache Spark
- Nov 26, 2020 - Added content